Research Article, Endocrinol Diabetes Res Vol: 8 Issue: 5
Incidence of New Onset Diabetes in COVID-19 Patients: A Follow up Study in Pakistan
Mehwish Iftikhar1*, Saba Zartash2, Amna Rizvi3, Muhammad Javed Ahmed4, Asifa Kamal5, Muhammad Asim Rana6 and Azhar Hussain7
1Department of Medicine, Khawaja Muhammad Safdar Medical College, Sialkot,Pakistan
2Department of Lahore University of Management Sciences, Lahore, Pakistan
3Department of Endocrinology and Metabolism, Avicenna Medical College,Lahore, Pakistan
4Department of Medicine, Fatima Jinnah Medical University, Lahore, Pakistan
5Department of Biostatistics, Lahore College for Woman University, Lahore,Pakistan
6Department Medicine, CMH Lahore Medical College, Lahore, Pakistan
7Department of Medicine, Baqai Medical University, Lahore, Pakistan
*Corresponding Author: Mehwish Iftikhar, Department of Medicine,Khawaja Muhammad Safdar Medical College, Sialkot, Pakistan, Tel: 3004291641; Email: drmehwish19@gmail.com
Received date: 23 February, 2022, Manuscript No. ECDR-22-55348;Editor assigned date: 25 February, 2022, PreQC No. ECDR-22-55348 (PQ);Reviewed date: 11 March, 2022, QC No. ECDR-22-55348;Revised date: 25 April, 2022, Manuscript No. ECDR-22-55348 (R);Published date: 02 May, 2022, DOI: 10.4172/2470-7570.1000320
Citation: Iftikhar M, Zartash S, Rizvi A, Ahmed MJ, Kamal A, et al. (2022) Incidence of New Onset Diabetes in COVID-19 Patients: A Follow up Study in Pakistan. Endocrinol Diabetes Res 8:5.
Abstract
Introduction: Coronavirus infections are mild, but fatal cases also befall. Lethal cases have occurred in patients with comorbidities specially diabetes mellitus that may have bidirectional relationship. The route of entry of coronavirus is Angiotensin-Converting Enzyme 2 (ACE2) receptors; these are expressed in key metabolic organs including pancreatic beta cells. Development of diabetes is via diverse etiologies. There is desperate need of research to see factors that cause new onset diabetes on a background of COVID-19.
Methods: In this follow up study 91 COVID positive patients of age 18-80 years were selected from COVID ICU via consecutive sampling after ethical approval and written informed consent. BMI, HbA1C and BSF were taken as baseline. Subjects with normal BSF and HbA1c were labelled normal, and selected for follow up. On two follow up visits done on 2nd and 6th month, BSF values were checked. Subjects with BSF more than 126 mg/dl were labelled as diabetic.
Results: Out of 91 patients, 69 (75.8%) were labelled 'diabetics' and 22 (24.2%) remained nondiabetic. These values were statistically significant with p-value < 0.05.
Conclusion: Sizable number of previously euglycemic patients developed new onset diabetes post COVID-19 infection in our study. This conclusion opens new horizon for research in terms of exploring underlying pathophysiology, contributory risk factors, transient or permanent nature of disease and finally management approaches with long term follow up goals of these newly diagnosed diabetes post COVID-19. Coordinated worldwide efforts are required to focus these concerns.
Keywords: COVID-19, New Onset Diabetes, HbA1C
Introduction
Coronaviruses are widely distributed in animals and humans. They are positively single stranded enveloped RNA viruses [1]. Though Coronavirus infections because mild symptoms but deadly outcomes have been documented too. Large outbreaks of two beta Coronaviruses that is Severe Acute Respiratory Syndrome Coronavirus (SARS-CoV) in 2002–2003 and Middle East Respiratory Syndrome Coronavirus (MERS-CoV) in 2012, have caused havoc that resulted in lot of mortalities [2,3].
In December 2019, pneumonia cases of unknown etiology emerged in Wuhan, Hubei Province, China. Deep sequencing analysis was carried out on the samples from lower respiratory tract. These came up with a new coronavirus as the underlying cause. It was named Severe Acute Respiratory Syndrome-Coronavirus-2 (SARS-CoV-2), and the disease it causes called COVID-19 [1,4]. Pathophysiology is still not completely understood it has been inferred that most severe cases with COVID-19 have occurred in the elderly or in patients with underlying comorbidities like cardiovascular mortalities, diabetes mellitus, chronic lung and renal disease, hypertension, and cancer [5,6].
Diabetes is considered to be the focal reason of morbidity and mortality throughout the world. This disease is associated with multiple complications including microvascular and macrovascular ones having significant impact on the overall patient’s survival [7]. It is a well-recognized fact that diabetics are more prone to various infections. It also influences the recovery time [8]. Virus are also known to induce autoimmunity for example infections by viruses that target beta cells and promote strong inflammation within the islets may thus represent the initial step in the induction of autoimmunity, Inflammation and autoimmunity are the etiological basis of diabetes [9]. The relationship between diabetes and COVID-19 may be bidirectional. On one hand, diabetes is associated with an increased risk of severe COVID-19. On the other hand, it has been observed that patients with COVID-19 develop new onset diabetes [10]. Severe Acute Respiratory Syndrome Coronavirus 2 (SARS-CoV-2), the virus that causes COVID-19, binds to Angiotensin-Converting Enzyme 2 (ACE2) receptors that are expressed in various organs and tissues like pancreatic beta cells, the small intestine, adipose tissue, and the kidneys [11]. Thus, it is probable that SARS-CoV-2 could complicate the pathophysiology of already existing diabetes or may lead to new mechanisms of disease by causing alterations in glucose metabolism. This is the reason that there is a desperate need for rapid good quality research into mechanisms responsible for its disease causing behaviors among humans. This would allow risk stratification and allocation of precious resources hereby.
Objective
To determine the incidence of new onset diabetes in COVID-19 patients.
Materials and Methods
The study was conducted at COVID-ICU Services Hospital Lahore. Total 91 COVID positive patients were selected through Convenient Sampling technique. It was a follow up study done from 1st June 2020 to 30 September 2020.
Methodology
After ethical approval, written informed consent was taken from patients themselves where possible, or their next of kin. Ninety-one (91) Adult patients with positive PCR by nasopharyngeal swab for COVID-19 and age ranging from18-80 years and having normal HbA1C were recruited from COVID ICU of Services Hospital Lahore. All Pregnant and lactating females and patients already on steroids for other reasons were excluded.
Patients were asked about history of hyperosmolar symptoms regarding Diabetes and their clinical parameters including BMI (kg/m²) and these were documented on a proforma in addition to blood glucose levels. Laboratory investigations were recorded included HbA1C, baseline fasting blood glucose (BSF) and random blood glucose (BSR) in addition to routine inflammatory profile for COVID-19 patients.
Those patients who did not have diabetes on baseline HbA1C, BSF and BSR were followed up. Patients who recovered and discharged were followed up after 2 month and 6 month (considering washout period of Steroids that was likely given to patients infected with COVID-19) readings of BSF were taken at 2 month and at 6 month after discharge and at 6th month HbA1C was also taken. Those with two deranged follows up BSF and HbA1C readings on follow up were labelled diabetic according to ADA criteria that is equal to or more than 126 mg/dl [12].
Statistical analysis
All information was noted down and data put in SPSS version 25, quantitative data like age were presented as means and standard deviation and qualitative data like gender was presented as frequency and percentages. Binary logistic regression analysis was carried out to determine the factors contributing to development of new onset diabetes.
Results
91 patients were analyzed by SPSS version 25. Out of 91 patients 69 (75.8%) developed diabetics and 22 (24.2%) remained non diabetics. From bivariate analysis it is observed that obesity (X2=6.912; p-Value=0.009), severity of disease (X2=15.994; p-Value=0.000064), baseline Ph (X2=5.197; p-Value=0.023), baseline Ferritin (X2=14.058; p-Value=0.007) TLC (X2=11.970; p-Value=0.003), LDH (X2=4.047; p-Value=0.044) and Antiviral treatment (X2=9.903; p-Value=0.002) are significantly associated(Table 1).
Table 1: Demographic characteristics and Lab parameters of population under study (n=91).
Factors with onset of diabetes. Ferritin is found only significant factor (OR=0.997; p-value=0.041) in binary logistic regression model when effect of all factors is studied simultaneously.
Our data shows out of 91 patients 52 (57.1%) were male, 39 (42.9 %) were female. It is depicted in Table â? that mean age of population studied was 59 years (± 11.02171), mean BMI (kg/m²) 28.69 (± 7.06909), mean baseline SpO2 (mmHg) 84.0659 (± 7.67797), mean pH 7.2 (± 0.12189), mean TLC 13.6 10^9/L (± 4.85082), mean lymphocyte count 2.8330 10^9/L (± 0.79331), mean Ferritin 880.6264 mcg/L (± 388.29324), mean LDH 426.3187 IU/L (± 308.44380), mean D-dimer mcg/ml 5.92 (± 4.73965), mean CRP 121.23 mg/L (± 68.49348), mean HbA1C (%) 5.5798 (± 0.42971) (Table 1).
Table 2 shows that among age group (18-40 years), 6 (85.7%) out of 7 developed new onset diabetes. Among 41-60 years, 32 (82.1%) out of 39 became diabetics and among 61-80 years, 31(68.9%) out of 45 became diabetic. Age has insignificant (X2=2.379; p-value=0.304) association with onset of diabetes. Among non-obese (BMI in kg/m²; 18-29.9), 44 (86.3%) people out of 51, became diabetic and among 40 Obese (BMI in kg/m² ≥ 30) patients, 25 (62.5%) people became diabetic, so BMI had significant association with onset of diabetes (X2=6.912; p-Value=0.009). Gender had insignificant relationship as out of 52 males 40 (76.9%) and 29 (74.4%) females out of 39 became diabetic (X2=0.080; p-Value=0.777).
Total of 11 (45.8%) patients out of 24 who had mild to moderate disease (SpO2 ≥ 90%) and 58 (86.5%) patients out of 67 who had severe disease (SpO2 ≤ 89%) developed Diabetes(X2 =0.000064; p-Value=15.994).
Among patients having Ferritin 11-337 mcg/L, 4 (57.1%), among patients having Ferritin 337-700 mcg/L, 13(54.2%) out of 24, among 700-1000 mcg/L 25 (78.1%) out of 32, among 1001-2000 mcg/L, 26(96.3%) out of 27 and among >2000 mcg/L, 1(100%) became Diabetic(X2=14.058; p-Value=0.007). Likewise, value of LDH at baseline had significant relationship as among those patients that had LDH 100-300 IU/L, 27 (29.7%) out of 41 (45.1%) and among those who had value of >300 IU/L, 42 (46.2%) out of 50 (54.9%) became diabetic(X2=4.047; p-Value=0.044). 53 (85.5%) patients out of 62 who received anti-viral treatment became diabetic however 16 (55.2%) patients out of 29 patients who did not received anti-viral treatment also became diabetic(X2=9.903;p-value0.002) (Table 2).
â?° Significant at 5%
Table 2: Stratification of new onset diabetes with different confounding variables (n=91).
Abbreviations: p stands for probability of rejecting null hypothesis when it is true.
Footnotes: Statistical significance for difference in proportions is calculated using Pearson’s Chi-Squared test. P less than 0.05 were considered statistically significant percentages are row wise.
Data are numbers and percentages (%) unless indicated otherwise. P value significant at 0.05 (Figure 1 and Table 3).
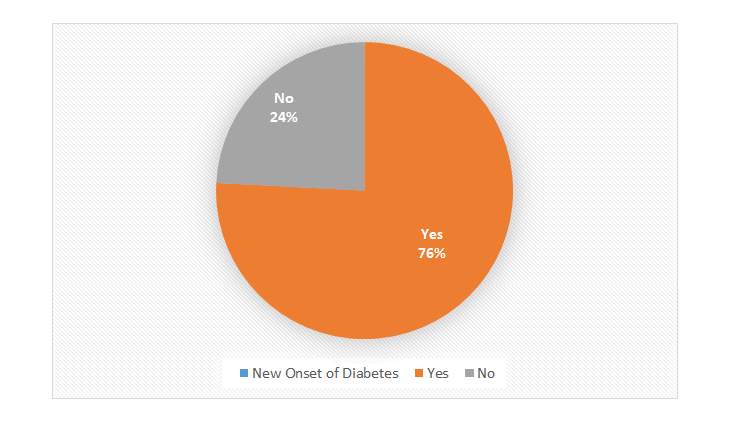
Figure 1: Percentage of new onset of diabetes in COVID-19 Patients.
Figure showing almost two third patients (75.78%) developed new onset Diabetes.
Table 3: Classification table for new onset of diabetes patients in COVID-19 patients.
Binary logistic regression analysis was performed to determine the risk factors associated with the development of diabetes in previously euglycemic patients who suffered from COVID-19 (Table 3). Development of diabetes is dichotomized as Yes/No and named as New Onset of Diabetes.
The outcome variable in current study was New Onset Diabetes. The potential risk factors included in the model were BMI, Gender, TLC, SpO2, Ferritin, CRP, Baseline HbA1C, Presence or absence of co-morbidities (excluding Diabetes) treatment in the form of antivirals and immunomodulation.
From Table 3, it is evident that the fitted Binary logistic regression model has 84.6% correct prediction hence it can be concluded that this model is appropriate to use for prediction to evaluate goodness of fit of model R^2 and Hosmer and Lemeshow test has been used. It can be seen that 41.4% of total variability in data is explained by fitted model. It can be concluded on the basis of Hosmer and Lomeshow test that current model is good fit to the data (Chi square=9.418, p-value=0.308).
BMI has positive impact (OR=1.088; p-value=0.085) on the onset of diabetes among COVID-19 patients but effect is observed insignificant. The risk of developing diabetes is increased by almost (1.088-1=0.088) 9% for per unit increase in the BMI holding effect of other factors constant. HbA1C has positive impact (OR=1.100; p-value=0.891) on the onset of diabetes among COVID-19 patients but effect is observed insignificant. The risk of developing diabetes is increased by almost (1.100-1=0.1) 10% for per unit increase in the HbA1C holding effect of other factors constant.
For gender, female is used as reference category. Chances of onset of diabetes in COVID-19 male patients are twice as compared to COVID-19 female patients assuming effect of rest of factors constant. Contribution of this factor in development of diabetes among COVID-19 patients is not significant (OR=1.999; p-value=0.343).
Chances of onset of diabetes is significantly decreased (OR=0.997; p-value=0.041) by 0.3% (1-.997=0.03) for per mcg/L increase in the ferritin keeping effect of other factors constant.
Chances of onset of diabetes is decreased (OR=0.995; p-value=0.415) by 0.3% (1-0.997=0.005) for per unit increase in the CRP keeping effect of other factors constant. SpO2 has positive impact (OR=1.126; p-value=0.051) on the onset of diabetes among COVID-19 patients.
Chances of onset of diabetes is significantly decreased (OR=0.954; p-value=0.595) by 4.6 % (1-.997=0.046) for per unit increase in the TLC keeping effect of other factors constant.
Regarding comorbidities, having no co morbidity is used as reference category. Chances of onset of diabetes in COVID-19 patients having co morbidities are 61% higher as compared to patients having co morbidities assuming effect of rest of factors constant. Contribution of this factor in development of diabetes among COVID-19 patients is not significant (OR=1.607; p-value=0.495).
Regarding Immune Modulation, Steroid treatment is used as reference category. Chances of onset of diabetes in COVID-19 patients who received steroids along with Tocilizumab are two third less as compared to patients who received Steroids alone assuming effect of rest of factors constant. Contribution of this factor in development of diabetes among COVID-19 patients is not significant (OR=0.347; p-value=0.446). Regarding use of anti-viral treatment, those who are not given Anti Virals are used as reference category. Chances of onset of diabetes in COVID-19 patients who did not received antiviral treatment are 14.6% higher as compared to patients who received anti virals having assuming effect of rest of factors constant. However, contribution of this factor in development of diabetes among COVID-19 patients is not significant (OR=1.146; p-value=0.852) (Table 4).
Table 4: ODD ratios for new onset of diabetes among covid-19 patients using binary regression model.
Discussion
In our Study 69 (75.8%) out of 91 patients who survived COVID-19 infection and stayed in ICU became diabetics and 22 (24.2%) remained non-diabetics. In our study severity of COVID-19 infection (SpO2< 89%) is observed to have a contributory role in developing new onset diabetes (p=0.000064). However, it is evident from descriptive that trend of recovered patients becoming diabetics was also seen in patients with increasing BMI (p=0.009) and those with raised baseline TLC count (p=0.03) and Ferritin (p=0.007). It is a well-known fact that inflammation is the basis of many chronic diseases like diabetes and even obesity [13]. Coronavirus induces a strong inflammatory response sometimes to the extent of cytokine syndrome [14]. Thus, It is inferred through our study that inflammation triggered by the virus may lead to new onset diabetes.
Diabetes is undoubtedly a multifactorial disease that is caused by combination of genetic and environmental factors [15]. Viruses remain major environmental candidates that carry known potential effects on pathogenesis of diabetes [16]. It is already established fact that there are many viruses which are affiliated with the onset of diabetes [17]. Similar observation is reinforced but for novel coronaviruses through our study results.
There are many trigging events that include several proposed mechanisms like auto-aggressive CD8+ T cells, together with other inflammatory cells and markers, infiltration of the pancreas leading to insulitis and hence destruction of the insulin-producing beta cells through autoimmune processes. Viruses play a pivotal role in induction of autoimmunity. Presentation of viral antigens by Antigen-Presenting Cells (APCs) activates the auto reactive T-cells and antiviral T-cells. In this way, molecular mimicry may augment autoimmune responses with the help of proinflammatory and inflammatory mediators [18]. These appealing mechanisms are yet to be proved in context of COVID-19. Our study is showing a promising start for unveiling more and more regarding this. Moreover, lysis of beta cells of pancreas by viruses leading to major insulin deficiency by destroying around 90% of pancreatic reserve may cause insulin requiring diabetes. However this is considered to be one of the mechanisms rather than the only key factor producing type 1 diabetes [19]. Still the role of direct viral cytopathic damage of pancreas cannot be discounted, and it seems that endocrine islet cells are particularly more vulnerable to the viral insult [20].
Twig G et al, showed in their work that WBC count, a commonly used and widely available test, is an independent risk factor for diabetes in young men [21]. Similar results are observed in our study in which COVID patients having increased TLC are more predisposed to have new onset diabetes (p=0.03) It is also evident from our results that higher ferritin concentrations are present in people who later became diabetic (p=0.007) [22]. As Lecube A et al, showed that diabetes is quite frequently associated with increased levels of serum ferritin. Moreover association of high serum ferritin levels with glucose intolerance, metabolic syndrome and insulin resistance in healthy people has also been recorded. An increase in body fat is associated with an increase in risk of metabolic diseases such as type 2 diabetes mellitus, hypertension and dyslipidemia. Similar finding regarding BMI is shown in our study as well (p=0.009) [23].
New-onset hyperglycemia is explained with COVID-19 in adults without a prior history of diabetes. Though several underlying pathophysiological mechanisms have been postulated but stress hyperglycemia cannot be ruled out in this overt stressful scenario of COVID-19 [24].
Conclusion
Significant number of previously non-diabetic patients developed new onset hyperglycemia post COVID-19 infection in our study. This conclusion opens new horizon for further research in terms of exploring underlying pathophysiology, contributory risk factors, transient or permanent nature of disease and finally management approaches with long term follow up goals of these newly diagnosed diabetes post COVID. Coordinated worldwide efforts are required to highlight these concerns.
References
- Yang X, Yu Y, Xu J, Shu H, Liu H, et al. (2020) Clinical course and outcomes of critically ill patients with SARS-CoV-2 pneumonia in Wuhan, China: a single-centered, retrospective, observational study. Lancet Respir Med 8:475-481.
- Song Z, Xu Y, Bao L, Zhang L, Yu P, et al. (2019) From SARS to MERS, thrusting coronaviruses into the spotlight. Viruses 11:59.
- Hussain A, Bhowmik B, do Vale Moreira N C (2020) COVID-19 and diabetes: Knowledge in progress. Diabetes Res Clin Pract 162:108142.
- World Health Organization(2020) Naming the coronavirus disease (COVID-19) and the virus that causes it. Department of Health and Family Welfare.
- Wu Z, McGoogan JM (2020) Characteristics of and important lessons from the coronavirus disease 2019 (COVID-19) outbreak in China: summary of a report of 72 314 cases from the Chinese Center for Disease Control and Prevention. Jama 323:1239-1242.
- Zhou F, Yu T, Du R, Fan G, Liu Y, et al. (2020) Clinical course and risk factors for mortality of adult inpatients with COVID-19 in Wuhan, China: a retrospective cohort study. Lancet 395:1054-1062.
- Williams R, Karuranga S, Malanda B, Saeedi P, Basit A, et al. (2020) Global and regional estimates and projections of diabetes-related health expenditure: Results from the International Diabetes Federation Diabetes Atlas. Diabetes Res Clin Pract 13:108072.
- Pearson-Stuttard J, Blundell S, Harris T, Cook DG, Critchley J (2016) Diabetes and infection: assessing the association with glycaemic control in population-based studies. Lancet Diabetes Endocrinol 4:148-58.
- Filippi CM, von Herrath MG (2008) viral trigger for type 1 diabetes: pros and cons. Diabetes 57:2863-2871.
- Chee YJ, Ng SJ, Yeoh E (2020) Diabetic ketoacidosis precipitated by Covid-19 in a patient with newly diagnosed diabetes mellitus. Diabetes Res Clin Pract 164:108166.
- Hamming I, Timens W, Bulthuis ML, Lely AT, Navis GV, et al. (2004) Tissue distribution of ACE2 protein, the functional receptor for SARS coronavirus. A first step in understanding SARS pathogenesis. J Pathol 203:631-637.
- American Diabetes Association (2020) Standards of Medical Care in Diabetes-2020 abridged for primary care providers. Clinical diabetes 38:10-38.
- Tsalamandris S, Antonopoulos AS, Oikonomou E, Papamikroulis GA, Vogiatzi G,et al. (2019) The role of inflammation in diabetes: current concepts and future perspectives. Eur Cardiol 14:50-59.
- Channappanavar R, Perlman S (2017) Pathogenic human coronavirus infections: causes and consequences of cytokine storm and immunopathology. Semin Immunopathol 39:529-539.
- Schneider DA, von Herrath MG (2014) Potential viral pathogenic mechanism in human type 1 diabetes. Diabetologia 57:2009-2018.
- Harris HF (1898) A case of diabetes mellitus quickly following mumps. Boston Med Surg J 140:465–469.
- Yoon J-W (1995) A new look at viruses in Type 1 diabetes. Diabetes Metab Rev 11:83–107.
- Filippi CM, von Herrath MG (2008) Viral trigger for type 1 diabetes: pros and cons. Diabetes 57:2863-2871.
- Rodriguez M, Garrett RS, Raitt M, Lampert PW, Oldstone MB. (1985) Virus persists in beta cells of islets of Langerhans and infection is associated with chemical manifestations of diabetes II. Morphologic observations. Am J Pathol 121:497.
- Boddu SK, Aurangabadkar G, Kuchay MS (2020) New onset diabetes, type 1 diabetes and COVID-19. Diabetes Metab Syndr Clin Res Rev 14:2211-2217.
- Twig G, Afek A, Shamiss A, Derazne E, Tzur D, et al. (2013) White blood cells count and incidence of type 2 diabetes in young men. Diabetes care 36:276-82.
- Lecube A, Hernández C, Genescà J, Esteban JI, Jardí R, et al. (2004) Diabetes is the main factor accounting for the high ferritin levels detected in chronic hepatitis C virus infection. Diabetes Care 27:2669-2675.
[Crossref] [Google Scholar] [Indexed]
- BaysHE, Chapman RH, Grandy S (2007) SHIELD Investigators’ Group. The relationship of body mass index to diabetes mellitus, hypertension and dyslipidaemia: comparison of data from two national surveys. Int J Clin Pract 61:737-747.
[Crossref] [Google Scholar] [Indexed]
- Ceriello A, De Nigris V, Prattichizzo F (2020) Why is hyperglycaemia worsening COVID 19 and its prognosis?Diabetes Obes Metab 22:1951-1952.